New Study Should Speed Discovery of Gene Function
By Jason Socrates
Bardi
Even though the complete and final sequence of the human
genome will be published next month, it should come as a shock
to nobody that we still have a long way to go in terms of
identifying and understanding how our genes actually function
in life.
In biology, this is referred to as the phenotype gapthe
discrepancy between the 30,000 to 40,000 genes we believe
are present in the human genome and the mere total of 5,000
distinguishable traits that have been identified through studies
of inherited diseases and knockout mutations produced by gene
targeting. The inference to be made is that the critical function
of most genes remains unknownwe know the genome sequence,
but we still have yet to discover all of its consequences.
Now a new study by a team of scientists, which was recently
published in the online edition of the journal Proceedings
of the National Academy of Sciences, could help to fill
in this gap, thanks to a team of scientists from the non-profit
Institute for Childhood and Neglected Diseases (ICND) at The
Scripps Research Institute (TSRI) in La Jolla; the non-profit
Genomics Institute of the Novartis Research Foundation; Phenomix
and Sequenom, Inc., both San Diego-based biotechnology companies;
the National Cancer Institute; and the Rockville, Maryland-based
company Celera Genomics.
The team conducted a massive study of the mouse genome to
examine the genomic variations within individual strains.
This analysis should help scientists decide which particular
mouse strains to breed in experiments aimed at mapping phenotypes
to genes, greatly increasing the speed with which the function
of genes are discovered.
"We now have a much clearer picture of the distribution
of DNA polymorphisms around the genome," says TSRI Assistant
Professor Colin Fletcher, one of the lead authors on the study.
Haplotype Patterns in Mouse Defined
Basically, explains TSRI Professor Steve Kay, the new tool
is like a global positioning system for mouse geneticsa
set of coordinates that scientists can use to "navigate" the
genome the same way that a sailboat pilot uses a constellation
of GPS satellites to navigate the Pacific Ocean.
The biological coordinates used in the new method are, in
actuality, what are known as single nucleotide polymorphisms
(SNPs). SNPs are locations in the genome where a particular
base can vary among individuals. Occasionally, these changes
occur in the middle of a gene and sometimes even alter the
function of the product of that gene, although the majority
of SNPs are not themselves responsible for disease.
Nevertheless, SNPs are extremely valuable for research if
they are located close to genes linked to a particular biological
traitlike susceptibility to a specific disease, for
instance. In these cases, the SNPs serve as biological markers
that scientists can use to identify and "positionally" clone
those linked genes.
In the study, the scientists analyzed the haplotypes, or
arrangements of these SNPs, throughout the mouse genome. In
fact, the team used technology developed by Sequenom, Inc.
and data generated by Celera Genomics to discover and identify
some 80,000 SNPs, a broad sampling of the mouse genome.
"We tried to get a set of SNPs that were spaced all over
the chromosomes," says GNF's Tim Wiltshire, who was the first
author on the paper.
What the team found was that the SNPs of the eight inbred
mouse species they surveyed tended to appear in clumps. That
is, certain regions had almost no SNPs, while other regions
were rich with the markers.
"You can go 50 million bases and find only a handful, and
then the next 20 million base pairs, there will be a SNP every
few hundred bases," says Fletcher.
This finding is intriguing because many scientists had assumed
SNPs were more or less randomly distributed throughout the
genome. But this analysis demonstrated that there is a structure
to the distribution of SNPs.
The findings also probably indicate something about the
nature of the laboratory micethat the ancestors of the
various inbred strains of mice were themselves inbred when
the strains were first established around the turn of the
last century.
Because of this inbreeding, no one strain can represent
the sum total of the biology of a mouse any more than one
family would represent the sum of all human biology.
Help for Cloning Genes
Based on this analysis of the haplotype patterns of the
different mouse strains, scientists should be able to compare
different strains of mice and determine which two would be
appropriate to breed in order to create a mouse model or attempt
to positionally clone a particular gene.
Positional cloning traditionally entails the use of classical
genetic mapping methods to confine the location of the gene
to a particular area in the genome, extensive sequencing of
the region in question, and the performance of computer-aided
searches through databases to find homology between sequences
in that region and known genes.
But with the new haplotype analysis and set of SNPs, researchers
could narrow the search dramatically. If a SNP is located
near a gene found to be associated with a particular trait,
scientists can recognize its proximity and use its known location
to identify nearby genes that may be linked to that trait.
Says Kay, "This [work] has allowed us to start cloning mouse
genes 100-fold faster than before."
To read the article "Genome-wide single-nucleotide polymorphism
analysis defines haplotype patterns in mouse" by Tim Wiltshire,
Mathew T. Pletcher, Serge Batalov, S. Whitney Barnes, Lisa
M. Tarantino, Michael P. Cooke, Hua Wu, Kevin Smylie, Andrey
Santrosyan, Neal G. Copeland, Nancy A. Jenkins, Francis Kalush,
Richard J. Mural, Richard J. Glynne, Steve A. Kay, Mark D.
Adams, and Colin F. Fletcher, see:
http://www.pnas.org/cgi/content/abstract/0130101100v1
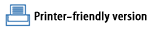
|