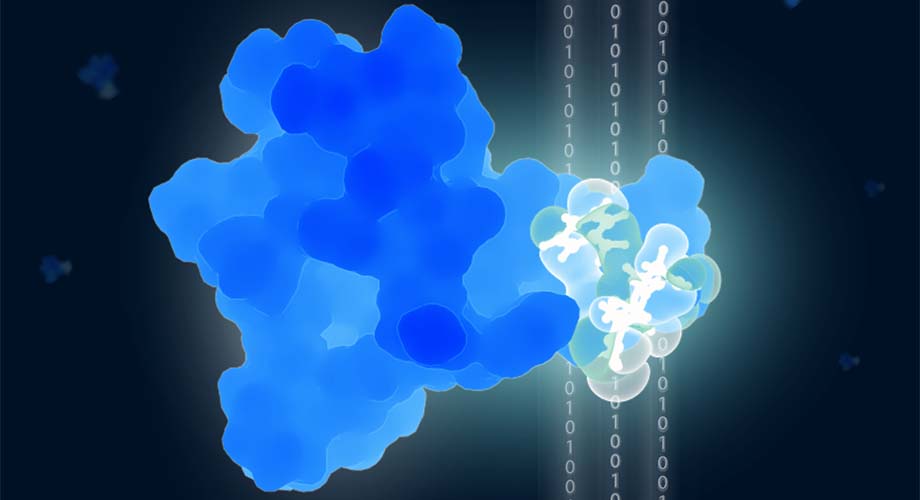
A protein molecule (blue) is used to study stabilizing interactions (white) between proteins and sugars. Scientists at Scripps Research have deepened our understanding of these vital interactions using quantum mechanics and artificial intelligence. (Illustration courtesy of the Kelly laboratory.)
When molecules collide: new method for analyzing sugar-protein interactions could enable better biologic drugs
The method, aimed at predicting how sugars stabilize proteins, should have broad applications in biology and medicine.
March 15, 2021
LA JOLLA, CA—Scientists at Scripps Research have found a new way to explain how small sugar molecules called glycans can help stabilize proteins that form the basis of certain medicines. The new approach represents a powerful tool for basic biochemical research and may pave the way to better, more affordable protein-based drugs.
Protein-based drugs are valuable weapons against a variety of diseases including cancers and autoimmune disorders. However, they are currently very expensive to develop and manufacture, and are often limited in their use because of their relative instability and short half-life in the bloodstream.
A natural process called glycosylation, in which glycans attach to proteins, occurs in essentially all animal cells and is one of nature’s ways of increasing protein stability. Biologists and drug designers would like to leverage this process as a general tool for engineering more stable, long-lived proteins—but on the whole, they have found it difficult to predict how a given glycan will affect its target protein.
In a study published in Nature Chemistry, the Scripps Research team developed a new and better way to make such predictions. Using an artificial intelligence technique called machine learning, along with quantum mechanics calculations, they showed how interactions of electrons within a protein and an attaching glycan help determine the degree of protein stabilization.
“It’s really a strategy that can be applied to all sorts of interactions between proteins and other molecules,” says the study’s first author Maziar Ardejani PhD, a senior staff scientist at Scripps Research.
Ardejani is a member of the laboratory of senior author Jeffery Kelly, PhD, the Lita Annenberg Hazen Professor of Chemistry at Scripps Research.
The team was guided by the “frontier molecular orbital” theory, which explains how electrons of molecules define their structures and interactions, Kelly notes.
“The field has struggled to predict glycosylation sites that would stabilize proteins,” he says. “This approach offers guidelines for achieving stabilization, which can have broad implications for drug development and beyond.”
Understanding stability
In the study, the researchers focused on a common type of glycan-to-protein attachment called N-glycosylation. In these attachments, the glycan bonds to a nitrogen atom (N) on an amino acid called an asparagine. Amino acids are the building blocks of proteins.
The researchers examined 52 pairs of closely related proteins, each pair consisting of an N-glycosylated and unglycosylated version of the same protein. They determined the electron-level structures of the interacting portions of the molecules and the resulting protein stability through experiments. Then they used an artificial intelligence approach known as machine learning to relate the two sets of data. The analysis yielded several rules, based on quantum mechanics, that clarify how N-glycosylation can be used to stabilize proteins.
These rules, the researchers say, represent a big improvement over existing, classical models of N-glycosylation’s effects, allowing them now to predict those effects more accurately—and making laborious, trial-and-error approaches less necessary.
Applications in pharmaceutics, biofuels and more
Ardejani expects that the method will be useful for designing N-glycosylated protein drugs with improved properties, including longer half-lives in the bloodstream, which enables drugs to work more effectively. The strategy, he adds, can also be used to design hyper-stable enzymes for biofuel conversion and for producing specialty chemical products.
The researchers see their approach as potentially relevant not only to protein glycosylation but to almost any instance in which molecules come together to form a more stable structure. They are currently working to extend their strategy to the analysis of interactions between proteins in living cells and the small organic molecules that make up most drugs.
“Stereoelectronic effects in stabilizing protein–N-glycan interactions revealed by experiment and machine learning” was co-authored by Maziar Ardejani, Louis Noodleman, Evan Powers and Jeffery Kelly.
The study was funded by the National Institutes of Health (GM51105, GM100934).
For more information, contact press@scripps.edu